Home
Mailgun Blog
IT & Engineering category
What you need to know about data processing for your infrastructure?
IT & Engineering
Data processing: methods in the madness
Data is everywhere. With a variety of data collection methods, storage options, and analysis protocols, how do you plan to decipher and use your data? We’ve got the scoop on the structural ins-and-outs of data processing. Trust us, it’s more dynamic than it sounds.
PUBLISHED ON
It’s a mad, mad world of data that we live in, but if you know how to harness its power, data can fuel everything from your SEO to your scalability. Understanding data processing methods is no picnic but we’ve got the guide – and you guessed it, the data – to break it down into byte-sized pieces. Pun intended.
Table of contents
Batch processing
Online processing
Time-sharing and real-time data processing
Data collection: Compliance and strategy
Structured data
Semi-structured data
Unstructured data
Six Vs of data analysis
Data centers and the cloud
Data storage and compliance
Table of contents
What is data?
Besides a conversation starter…
Data is information (graphs, facts, and statistics) generated by actions and transactions we make online. Try walking into an office, joining a Zoom call, or even hitting your local bar without data coming up in conversation. You might hear “I swear my smartphone is listening to me,” or “did you hear about the new compliance legislation?”
Don’t freak out. This is not a post about sentient phones or data rights (but if you’re interested, check out our posts on the GDPR and CCPA). This blog is about how to manage and process the data that runs your business.
What is data processing?
Data processing turns information collected from a variety of sources into usable information. When we talk about data processing we’re mainly talking about electronic data processing which uses machine learning, algorithms, and statistical models to turn raw data into a format that can be read by machines so that it can be easily processed and manipulated for forecasting and reporting by humans.
Remember elementary school, when you learned about reduce, reuse, recycle? Well, data processing is like recycling. Data is collected and “reduced” or broken down and processed for machine interpretation. Then, it is “reused,” or analyzed and stored for human interpretation and application. Finally, it’s “recycled”, or stored in an active database so it can be used to build more accurate projections and applications.
To fully understand the logistics of data processing, you have to view it almost as a natural resource rather than just collected information.
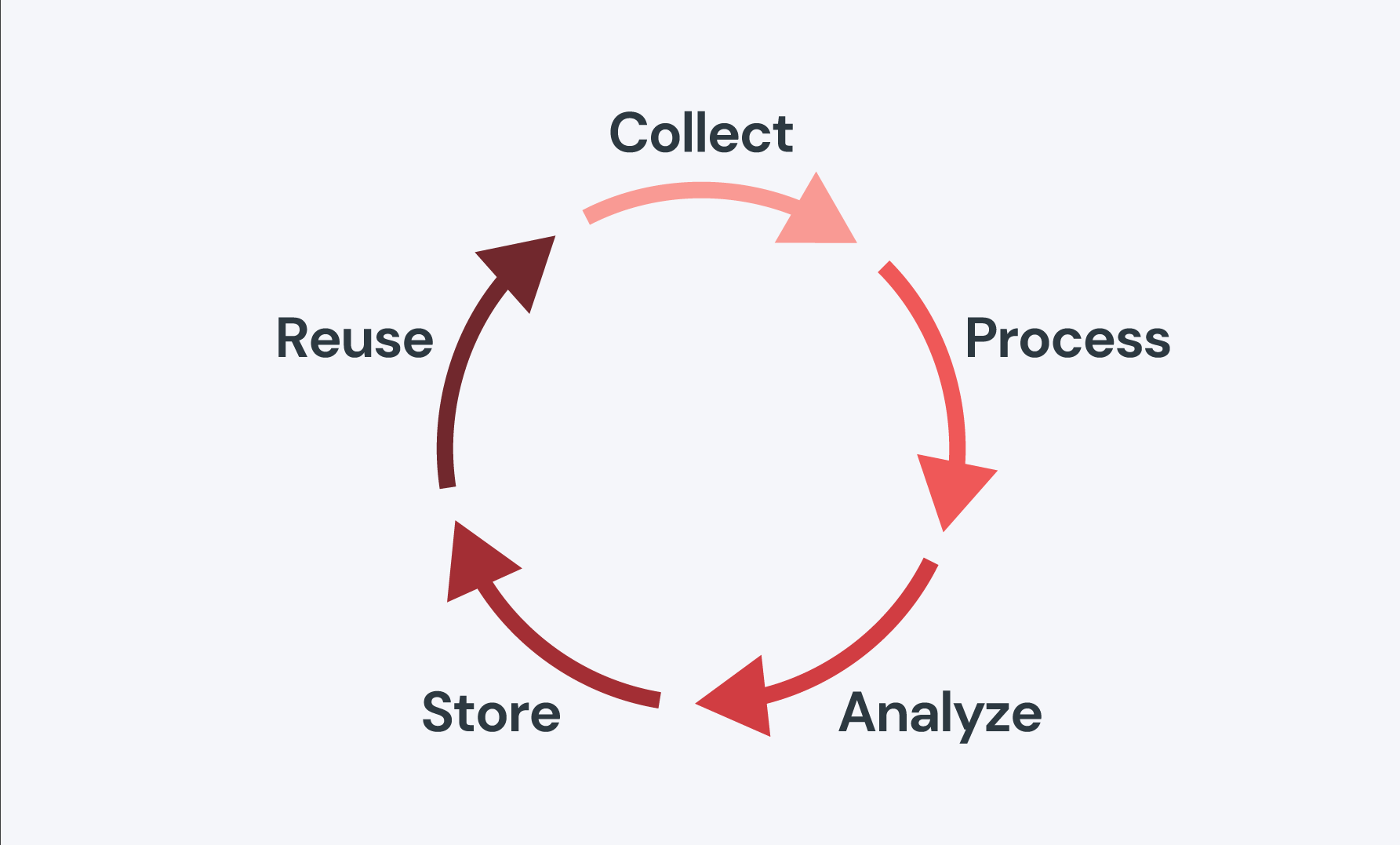
Types of data processing
Data can be processed in a variety of ways using different algorithms. In fact, you’re really only limited by the capacity of your infrastructure. Let’s take a quick look at the top three ways to process data.
Batch processing
In batch processing, your data is collected and processed in batches. This is the cookie analogy – you can make a giant batter of cookie dough, but you can only cook them in the batch sizes that will fit in your oven.
Have multiple ovens? Great, that means you can process more batches faster. Batch processing is best for really large amounts of data.
Online processing
With online processing, your data feeds directly into your CPU making it the preferred method of processing for data that needs to be available immediately – like if you’re checking out at the grocery store and the cashier is scanning bar codes to recall your items in their system.
Time-sharing and real-time data processing
A simple way to look at the time-sharing method is to think of a vacation timeshare where the resource (the vacation property) is split between multiple families. This method, also known as parallel processing, works by breaking data into small amounts and processing it between multiple CPUs either in parallel or consecutively.
Real-time processing is similar to timesharing in how data is processed. The main difference is how time is interpreted. Real-time processing provides data with very short latency periods (usually milliseconds) to a single application, while time-sharing deals with several applications.
What is data collection?
Data collection is data entry performed by your users. Before you can start processing, you have to get your hands on enough data to make an impact.
There are a lot of ways to collect data and you can (and should) use multiple collection points. Data comes from internet transactions, interactions, observations, and monitoring. Here are a few common methods:
Method | Description |
---|---|
Method | |
Transactional tracking | Data captured after an event like an online purchase, form submission, or password reset request. |
Description | |
Online tracking | Analysis of the behavior of online users i.e., browser tracking, web tracking, cookies. |
Surveys and interviews | Data collected by active/intentional user participation. |
Observational | How people interact with your product/site. |
Data collection: Compliance and strategy
In general, data compliance is a very political issue in terms of protecting consumers against data mining and other privacy violations. When it comes to data collection, the point in which the data is collected is important. For example, we recommend always growing your contact lists organically over purchasing them. Not only for the quality of the addresses, but for your sender reputation.
The point of collection can also have a lot of compliance legislation surrounding it regarding disclaimers and data policies you need to provide to potential users at the beginning of their journey.
In terms of data collection strategies, you want to approach data collection with the mindset of capturing the most useful and active data that you can. What do we mean? The point of collection is where you can involve users and add Captcha, require confirmation emails, verification codes via SMS, etc. to avoid accumulating a large amount of bot data that won’t benefit you.
Types of data
Not all the data you collect is going to be names and emails. We can break data down into three general types: structured, semi-structured, and unstructured.
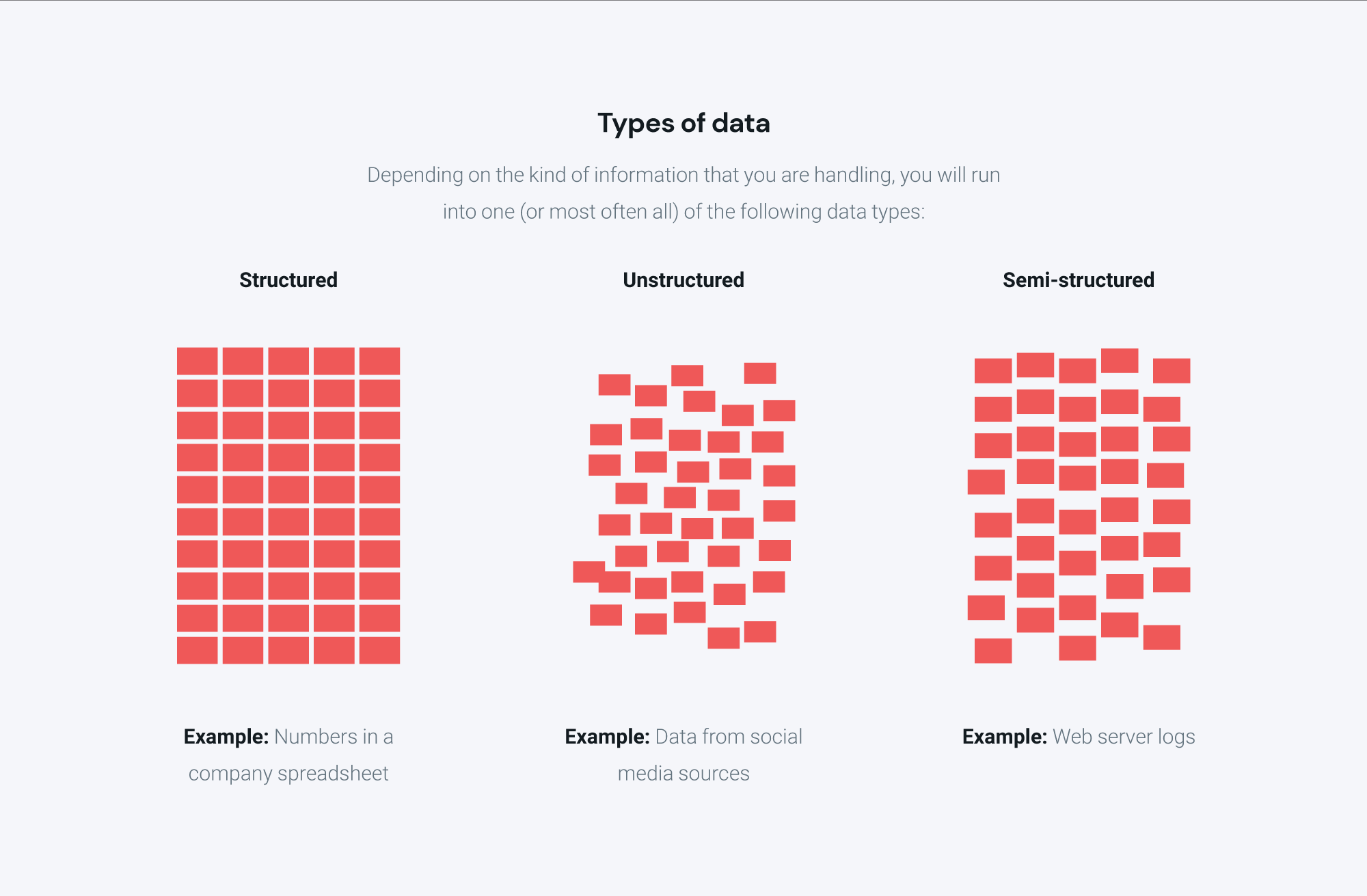
Structured data
Structured data is formatted to be easily understood by machine language. It is highly formatted and organized repository or dedicated database like My SQL or something similar.
Structured data is incredibly specific.
Formats for structured data are pre-defined using schema-on-write.
Fields can support a variety of information from a name to geolocational data.
Semi-structured data
Semi-structured data is organized but not in a relational database (one or more interrelated tables/rows.)
Semi-structured data has tags and is categorized or organized but it is not classified under a particular database. In other words, semi-structured data doesn’t conform to any one schema, or data format. Semi-structured data can be integrated from many sources, anything from zipped files to TCP packets or XML.
Unstructured data
Unstructured data has no organization or data model and can come in a variety of formats like images, text, or media.
Unstructured data has no predetermined format. Let’s say that structured data is like a roll of pennies, and only pennies can be collected because only pennies fit into the format. Unstructured data is the random loose change in your car. Anything from social media surveys to text files, audio, video, or images applies.
Where structured data uses schema-on-write, unstructured uses schema-on-read which means that data formatting is done on analysis for an individual set of data because the collection of the data is more important than how it’s organized. If we translate this to our coin example, all the coins can be quantified but you must first determine if you’re going to define them by color, value, size, etc.
What is data analysis?
Making a decision with some data is better than decision-making with no data at all. The first step to using your data is data preparation. In order to use the data you have collected, it needs to be machine-readable and then analyzed.
We’ve already talked about the different types of data structure, but what will different data aspects reveal?
Six Vs of data analysis
Data analytics is the processing of taking big data and breaking it down into a readable format so you can apply the benefits of the data to your business ventures and projections. There are a lot of ways to interpret data, so it helps to break down your analysis into these six segments.
Type | Description |
---|---|
Type | |
VOLUME: Volume is about scalability. | Volume forces you to answer one big question, how much data are you capable of processing? Collecting data is one thing, but when we talk about volume what we’re really talking about is the processing power of your infrastructure. How much data can you store, and how much data can you manipulate at any given moment? |
Description | |
VELOCITY: Velocity is about defining the conditions for processing your data within moments to get the results you need. | Velocity entails how fast your data is being received, such as in real-time or in batched quantities. Data is in a constant state of flux, and it becomes important to be able to process different types of data (structured/unstructured) quickly in order to seize geolocational opportunities and take advantage of real-time trends. |
VARIETY: Variety is about how your data is collected influences how it can be analyzed. | Variety speaks to the diversity of your data; where it came from, the value of the data, whether it was obtained from individual users or came from a larger enterprise source, etc. In terms of analysis, variety deals with how different data is standardized and distributed after you’ve collected it. |
VERACITY: Veracity is about the quality of the origin of your data. | How accurate is your data? Or, more importantly, what is the quality of the origin of your data. Veracity calls back your data collection process and the factors you have in place to ensure the data is high quality user data vs. bot data or disposable emails, etc. |
VALUE: Value is about usability. What are the applications for your data? | Determining the value of data is subjective. One way is to link the contribution of the data to how it affects your bottom line. Another is to value it by its usability – does your data have practical applications across initiatives or serve as a valuable resource? |
VARIABILITY: Variability is about building a baseline to compare one set of data to another for analysis. | What is the range of your data? Variability is how spread apart your collected data points are. Variance in a data set determines the range of the data collected (from smallest to largest points), as well as the deviation or how tightly your data points are clustered together around the average of the points. |
Storing data
Storing data doesn’t just mean that you collect it and throw it in a box in a digital basement somewhere for later use. Stored data is recorded data, processed so you can retain it on a computer or other device. Storing data also means you are capturing it in order to make it accessible. What’s the point in collecting data if you can’t apply it?
Data centers and the cloud
You have options when it comes to where you store your data. Cloud vs. on premise is its own debate, regardless of whether you’re looking at security or at data storage. In terms of data processing, you can either house your own data storage or you can store on hosted cloud solutions that utilize larger off-site data warehouses.
Data is alive. Ok, not actually alive, but data does grow as it’s collected. And the more data you have, the more powerful your applications can be. So, when you’re thinking about storage it’s a good idea to keep scalability in mind – and that tends to be simpler and more cost-effective with cloud-based solutions.
Data storage and compliance
Possibly the biggest hurdle for data processing as it evolves is secure storage and responsible use. Data compliance legislation is evolving quickly. One way to keep up with the policies is to opt for cloud-based infrastructure solutions that are built to scale as laws change – otherwise you’re on the hook to spend the money to update your own infrastructure.
While the U.S. doesn’t have federal data policies yet, states like California are starting to implement them with legislation like the CCPA. And let’s face it, many businesses operate globally and have to factor in European legislations like the GDPR into their policies.
Processing your data and making it accessible is only half the battle. You can’t use it if it’s not compliant. Curious how Mailgun manages your data? Check out our email and security compliance ebook for all the details.
Applying your data
Data processing is the playbook for making your data usable. Once your data has been evaluated and analyzed by machines, you can apply the data output to your business ventures. Use data to project market trends, user behaviors, and strategies performance improvements.
In our world, the world of email, data helps us offer features like Email validation that help you validate your emails addresses against our database to catch spam domains, typos, and other inconsistencies with incredible accuracy.
Like we said, data is a natural resource, and it can seem like an unlimited one. Data will likely not run out. As long as people interact online, data will remain a powerful tool, but without proper processing and effective data storage, your data is dead in the water. If you can’t manage your data, you can’t apply it.
Mailgun’s knowledge database
We can’t think of a subject bigger than data, or one that’s more interesting. Data informs everything from your email deliverability to your policies and information systems. It’s a giant topic and our team talks about it a lot.
Join our conversation and subscribe to our newsletter so you don’t miss out on insights and guides like this one.